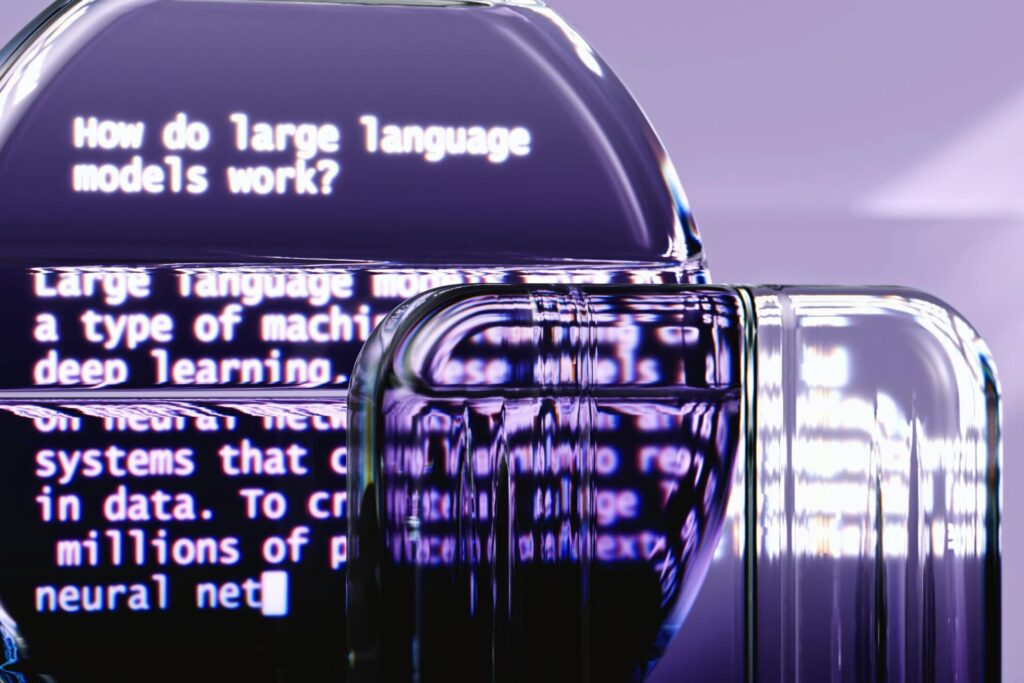
In the fast-evolving world of generative AI (GenAI), organisations are grappling with both promise and peril. While the technology has captured imaginations and budgets alike, the reality is sobering: through 2025, Gartner estimates at least 50% of GenAI projects are expected to be abandoned after proof of concept due to poor data quality, inadequate risk controls, escalating costs, or unclear business value.
Whether you’re a CIO, CTO or CDAO navigating technology investments or a business leader seeking ROI, understanding the root causes of failure is critical to making GenAI a cornerstone of your digital strategy.
The current landscape: Data at the heart of transformation
The rapid adoption of GenAI mirrors earlier technology waves, from graphical interfaces to mobile computing. Yet unlike those earlier shifts, GenAI’s success hinges on a single foundational element: data. High-quality, accessible, and well-governed data isn’t just a nice-to-have — it’s the linchpin of GenAI’s ability to deliver meaningful results.
However, many organisations underestimate the complexity of preparing their data ecosystems for GenAI. Missteps at this stage not only undermine individual projects but can erode trust in AI initiatives across the enterprise.
Three key reasons GenAI projects fail
- GenAI doesn’t deliver business value
Too often, companies pursue AI for AI’s sake, prioritising technical novelty over business impact. Without clear alignment to strategic goals, even the most advanced GenAI models will fail to justify their costs.
The fix can be made by looking at business metrics. Identify use cases where AI can make measurable improvements to efficiency, revenue, or customer satisfaction. Communicate these impacts clearly and frequently to stakeholders, maintaining alignment between technical teams and business leaders.
- GenAI alone isn’t the right technique
GenAI is powerful, but it’s not a silver bullet. Many successful AI initiatives use a composite approach — combining generative techniques with predictive analytics, machine learning, or rule-based systems — to solve complex problems.
Adopting a composable AI strategy can leverage the strengths of multiple AI techniques, ensuring flexibility and robustness as your organisation’s needs evolve.
- AI is ready, but the data isn’t
Even the most sophisticated AI systems depend on the quality of their data inputs. Fragmented, siloed, or biased data sets often doom projects before they begin.
Start by investing in foundational data readiness. Equip teams with the skills and tools to prepare data pipelines, automate chunking, apply vector embeddings, and fine-tune models efficiently. Building a robust data governance framework ensures scalability and reliability.
Lessons from the field: Successful strategies
For organisations that have navigated the complexities of GenAI, the journey offers valuable insights.
Embedding AI Literacy stands out as a foundational step. Some companies have reimagined AI adoption as a cultural transformation, equipping their workforce with the knowledge to make informed decisions. By embedding AI education into the organisational DNA, companies empower employees to assess not only the opportunities of GenAI but also its financial and operational implications. The result is a workforce prepared to collaborate effectively on AI initiatives, minimising the friction often seen between technical and business teams.
Fostering Safe Experimentation is another critical practice. At institutions like Harvard, AI sandboxes provide a controlled environment where teams can explore the potential of GenAI without exposing the organisation to undue risk. These sandboxes combine cutting-edge tools for data analysis, visualisation, and experimentation, ensuring that innovation can flourish within defined boundaries. Such environments also serve as incubators for best practices, as teams refine their approaches before scaling solutions enterprise-wide.
Structuring for Success involves more than deploying technology — it’s about creating the right organisational framework to sustain AI initiatives. Financial services firms exemplify this with their dedicated AI oversight and vendor management teams. By clearly delineating responsibilities and fostering cross-functional collaboration, organisations can sidestep common pitfalls like scope creep and misaligned expectations.
These examples illustrate that success in GenAI requires more than technical innovation. It demands a holistic approach that weaves cultural, structural, and operational readiness into the fabric of the company. By investing in education, creating safe spaces for experimentation, and building robust organisational frameworks, businesses can turn the promise of GenAI into measurable impact.
What’s at stake: The cost of failure
The consequences of failed GenAI projects extend beyond wasted resources. Each setback diminishes confidence in AI initiatives, making it harder to secure buy-in for future efforts. Meanwhile, competitors that master these challenges will not only save costs but also unlock transformative value—widening the competitive gap.
Yet, the cost of inaction is even greater. As businesses increasingly rely on data and analytics for strategic decision-making, those without effective AI strategies risk irrelevance in a rapidly digitising economy.
A call to action
Realising GenAI’s potential requires more than technical expertise. Success depends on aligning AI initiatives with business goals, adopting flexible architectures, and addressing foundational data challenges.
As organisations forge ahead, the imperative is clear: treat data as a strategic asset, foster cross-functional collaboration, and prioritise education at all levels of the organisation. With the right strategies, the 50% failure rate predicted today could become a 100% success rate tomorrow.
Gartner analysts will further explore how data shapes AI and how to harness the power of GenAI at the Gartner Data & Analytics Summit in London this week. The conference will delve into strategies for overcoming data readiness challenges, scaling AI effectively, and driving tangible business outcomes in an AI-driven world.
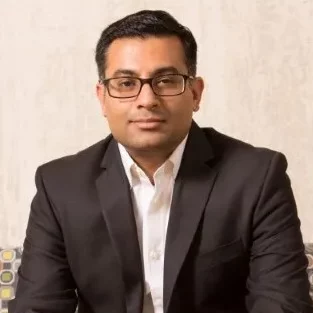
Soyeb Barot
Soyeb Barot is Distinguished VP Analyst at Gartner.