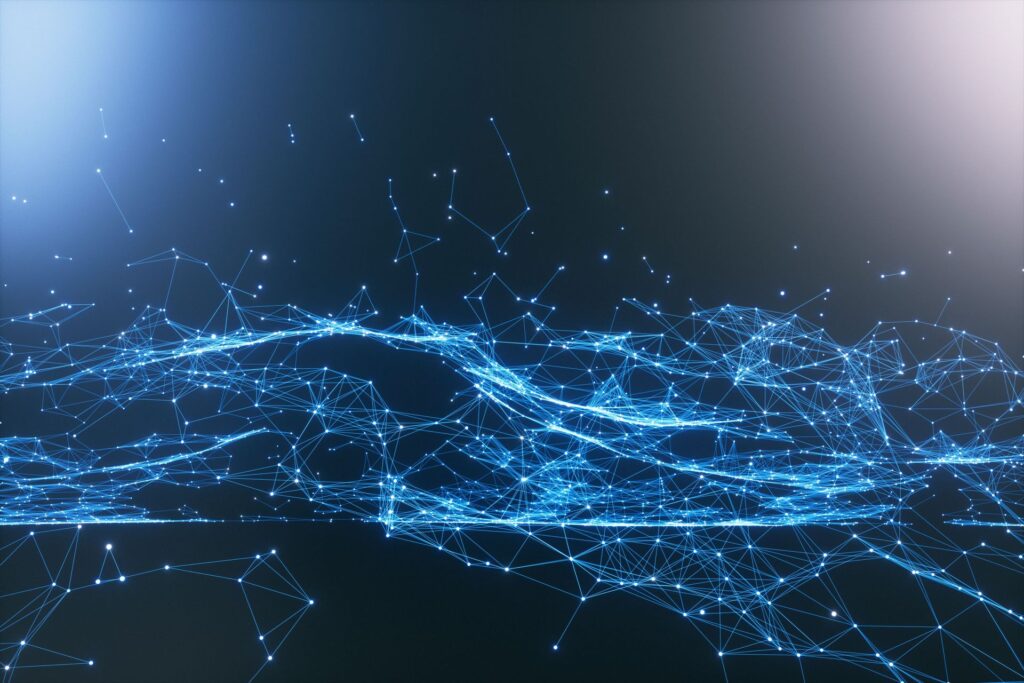
The hype around Artificial intelligence (AI) shows no signs of abating anytime soon. In fact, hype seems an apt description given the publication earlier this year of the Gartner Hype Cycle for AI, 2019, the results of which reflect the growing popularity of enterprises ramping up AI adoption.
It seems as if everyone wants to talk about AI and everyone wants to apply intelligence for better insights and better decisions. According to Gartner, the number of organisations that have deployed AI grew from 4% to 14% between 2018 and 2019, while global business value derived from AI is forecast to reach $3.9 trillion in 2022.
In October 2019, it was also announced that the world’s first graduate level, research-based AI university would open in 2020. The Mohamed bin Zayed University of Artificial Intelligence has already received over 3,000 applications as it promises to provide students with access to some of the most advanced AI systems in existence.
However, the pursuit of AI is not new. In fact, it can be traced back to the days of Alan Turing and the ‘Turing Test’ developed in 1950, which is widely regarded as the foundation of the philosophy of artificial intelligence by many.
What has changed, however, is the attention being given to AI today. More and more companies are forming in the market, thanks in no small part to significant investment and research from the likes of Google and Amazon in particular. For example, researchers at Google announced in October 2019 that they have taught an AI machine how to smell by training it to accurately distinguish and categorise different smells based on assessing their molecular structure.
The excitement around AI is easy to grasp given its powerful capabilities: applying intelligence against data to gain better insights and make better decisions. After all, this is what the human brain is designed to do all of the time.
However, there’s generally always something missing from all of these discussions. That of the quality of the data being used. Artificial intelligence – in fact, any intelligence – can only be as good as the data from which it is drawing inferences.
The fact is that the data owned and stored by businesses, government departments and other major institutions today is too often in a complete mess, constrained by the silos it’s stuck in that have been built over the years as organisations grow. When you factor in mergers, acquisitions and the amalgamation of legacy and new IT systems, the number of data silos only increases.
For many companies, the state of their data is similar to an attic full of overflowing boxes, each one different and full of ‘stuff’. Now consider that ‘stuff’ – a quarter of which is valuable, a quarter of which is useless, a quarter of which should probably be stored elsewhere, and a quarter that everybody has likely forgotten about or doesn’t even know is there.
Getting all of this ‘stuff’ into one AI engine is a challenge, but the preparatory work required to do this in terms of consolidating the data in order to get an integrated view with lineage and governance also in place is crucial if AI is to realise its full potential. In short, an AI engine is only going to be as smart as the quality of the data put into it, and so data quality must become more of a focus for companies.
However, not only is data all too often stuck in silos across enterprises, which makes integrating it difficult and expensive, but data scientists continue to report the majority of their time is spent on ‘data wrangling’ instead of analysis. Based on interviews and estimates, up to 80% of a data scientist’s time is spent on the work they like the least – collecting, labelling, cleaning and organising data – in order to get it into a usable form for analysis.
This problem won’t subside with the adoption of AI technologies. AI needs data that is clean, up-to-date and well governed so everyone accessing it has a 360-degree view of where it came from, how it was collected and how it might have been transformed before getting to an AI engine.
The same is true for compliance, with legislation such as the General Data Protection Regulation (GDPR) and California Consumer Privacy Act (CCPA) requiring organisations to have a comprehensive view of their data with unified governance, knowledge of the history of the data and good controls.
AI offers many advantages, but amidst the marketing drive and endless thirst for AI technologies, it would be easy to forget the importance of security. While AI relies on data that is easily accessible and shareable, privacy concerns, particularly around consumer data, will only continue to grow as more intelligence is set against data, revealing connections that may infringe on privacy boundaries. Rigid security and visibility controls as well as data governance are therefore critical.
What’s clear is that if artificial intelligence truly is the key for increased efficiency across all types of businesses, then good data is the vital ingredient.
David Northmore, VP EMEA, MarkLogic