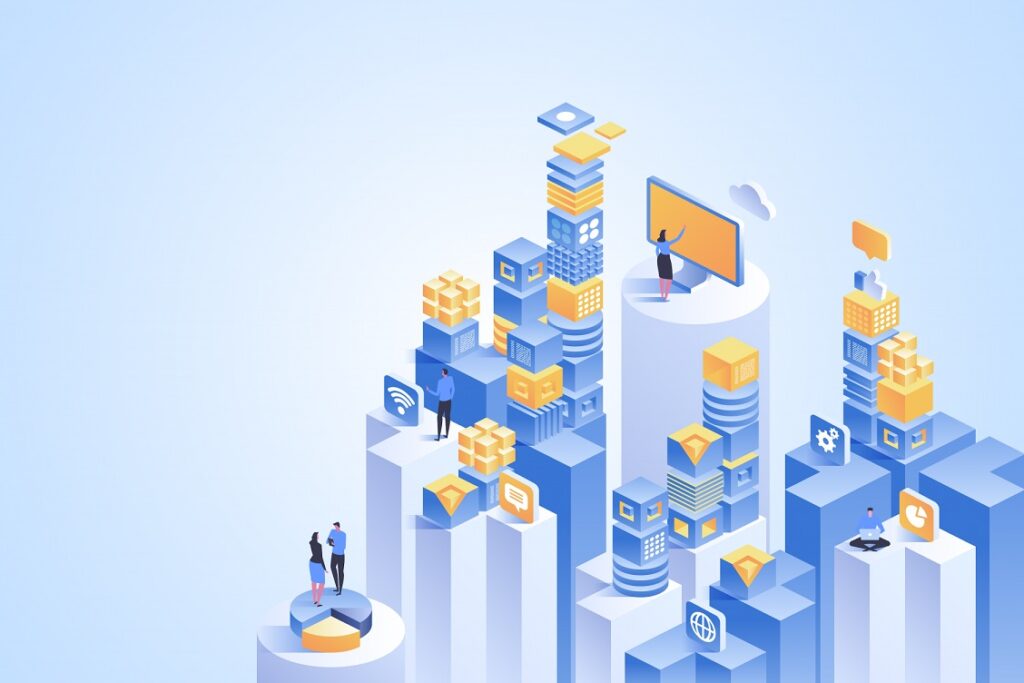
A recent report from Gartner looking at the major concerns of CEOs will have made for uncomfortable reading for their CIO counterparts. It found that next to concerns over globalisation and economic issues – in a pre-COVID-19 world, it is worth pointing out – “digital dithering” was focusing the minds of boards of directors and Chief Execs.
Some digital programmes are almost a decade old – the report said many started between 2011 and 2013 – with hundreds of billions of dollars invested in digital platforms and infrastructure to enable lasting transformations. There is now an expectation that such significant outlays start showing tangible results.
But many companies are effectively working with one or even both their arms tied behind their back when it comes to digital transformation programmes because of an inability to properly treat their data. That’s according to Jonathan Bowl, Hitachi Vantara’s VP & GM, Big Data & IoT, who tells Digital Bulletin that many businesses have, and will continue to, fall by the wayside as a result.
“There is a phrase that I use that is “Digital Darwinism’, and I think that since 2000, 52% of the FTSE 500 companies at that time no longer exist as they were then,” he says. “They’ve gone out of business or been acquired and what we are seeing now is the survival of the fittest. Businesses that are transforming and modernising are the ones that are keeping pace.
“We engage with a significant number of organisations and one of the first challenges we come across is finding the data. Large enterprises will tell us that they have a lot of it but that they don’t know who owns it or who is responsible for it.
“Most companies have grown over time in silos, so IT projects have been driven on a departmental basis, you have financial systems, CRM systems for sales, and operational systems. Each department has its own budget and has built up silos of data. If you are a siloed organisation, you are as far away from digital transformation as it is possible to be.”
Hitachi Vantara, and Bowl in particular, are vocal proponents of DataOps – combining data and operations – which has been billed as “data management for the AI era”. DataOps, deployed correctly, has the power to realise data’s ultimate potential by automating the processes that enable the right data to get to the right place at the right time, while ensuring it remains secure and accessible to authorised employees across the enterprise.
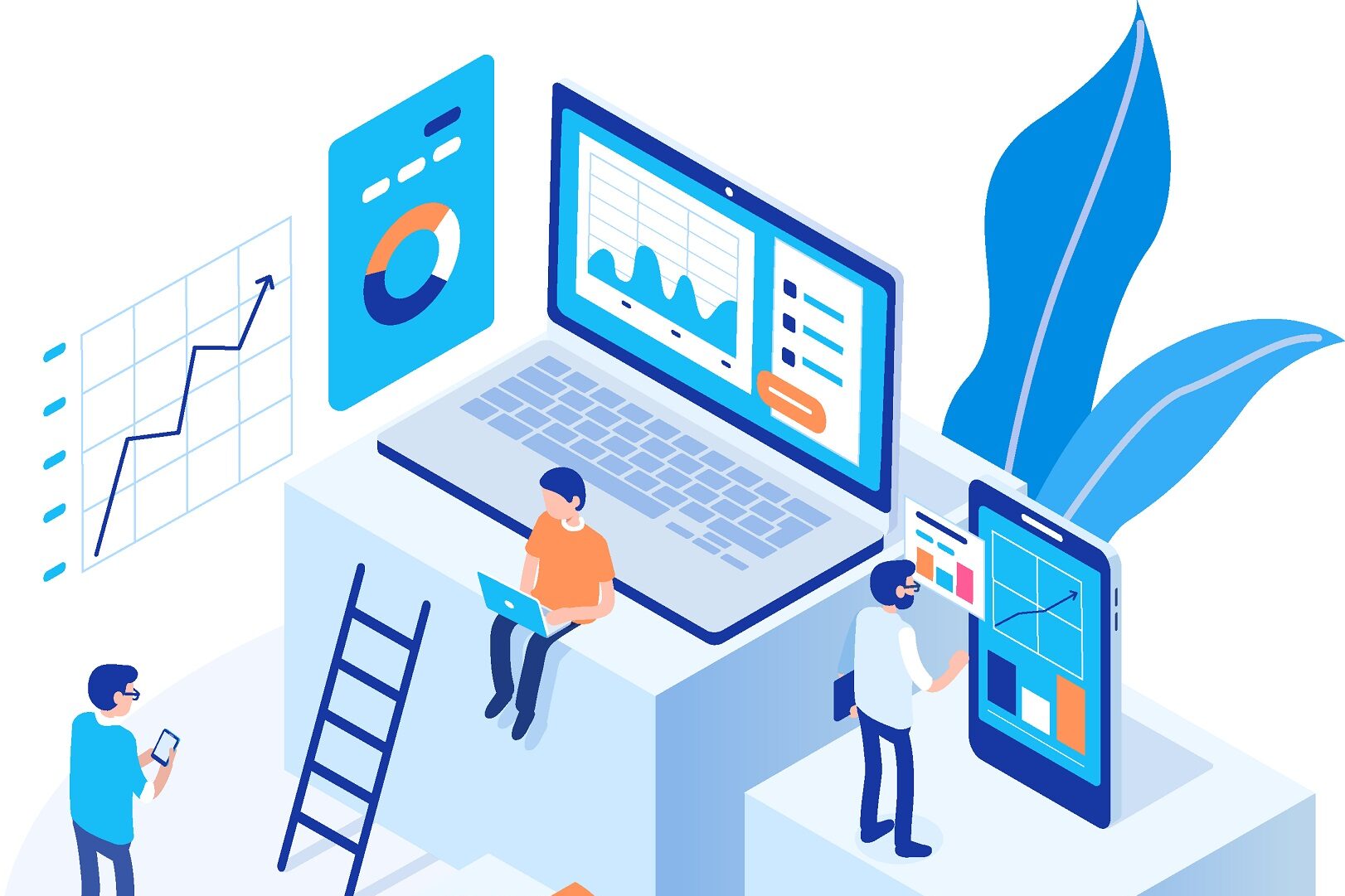
The volume around data and its value has never been louder, but companies are still coming up against the same issues: human-dependent data collection, duplicated data, inaccurate data and “dirty data” have all been cited as huge roadblocks to transformation. Bowl says that a tendency to look at data exclusively in the present tense is also proving problematic and stopping companies from unlocking valuable insights.
“If you look at a big data maturity model, organisations are using their data to understand what happens today: how many people came into the store, how many products did I sell? But few organisations are using their data to power their business in order to become more predictive and prescriptive and to transform their business.
“Our job is trying to help organisations with the data that they’ve got to accelerate through the big data maturity curve in order to become more predictive, more prescriptive and build those data models that help them understand the variables and the metrics that are better indicators of performance. This helps with service and engaging with customers in new and meaningful ways.”
Hitachi Vantara works with many big enterprise players across the industry spectrum, and comes into its own, according to Bowl, when it is tasked with answering truly challenging data questions.
Clients include the likes of Disney, Nasdaq and NASA, while it also works with other business units in the Hitachi ecosystem, such as Hitachi Rail, where it has built neural networks to take information from thousands of data points on rolling stock to predict issues and pain points.
“Organisations have tonnes of data that they don’t need. When you start talking about real-time sensor data, after a matter of moments it is no longer valuable, it has gone past its usefulness,” says Bowl.
“We’re working with Hitachi Consulting and other partners to build a platform that helps the rolling stock to predict when something is going to fail and provides all the necessary contexts. For example, just being told a door is open has no consequence, but if you’re being told a train is going 100mph and the door is open, then that is something to act on. That’s a really good example of where we are using a lot of our assets, both physical and IT, to answer important questions.”
The company has also worked with Stena Line to introduce what it says is the first AI-assisted vessel. The model – known as the AI Captain – simulates different scenarios before suggesting optimal route and performance setup and is able to consider a number of variables, such as currents, weather conditions, shallow water and speed through water.
“We’re using the knowledge of the people on the vessels together with the neural networks that we’ve been building in order to optimise the ships. Neural networks are able to learn over time and what we’ve been able to do is reduce their fuel costs significantly by optimising the vessels using these data points in real time.”
Data is so powerful that it doesn’t need a metaphor and the reality is that it isn’t like oil, data does not deplete, it does not wear out
One phrase that is used a lot is ‘data is the new oil’, or the new sun or the new gold, the new currency, there are so many metaphors, and Bowl reckons to have heard them all. But there are some key differences that he says makes data stand out on its own as a resource.
“Data is so powerful that it doesn’t need a metaphor and the reality is that it isn’t like oil, data does not deplete, it does not wear out. It can be used for an infinite amount of time over and over again and the more you use your data, the more curated it becomes, which means there is more metadata, more indexing, which in turn makes it more valuable.
“The key to DataOps and the key to data engineering will be how you make all of this data available to everybody, because the more you use it the cleaner and more valuable it becomes. That is going to be really critical for organisations that want to use data science to find those better indicators of performance.”
But as with many areas of enterprise technology, the skills gap and a wider lack of data literacy are combining in a most unwelcome way. He cites a recent study that found almost two thirds of professionals would override decisions made by data platforms based on their gut feeling.
“That lack of data literacy and finding the right skills and people is going to be a major challenge. CIOs are under a lot of pressure so being able to hire people that can interpret data and what their company needs to do to transform and stay competitive is absolutely key. Data scientists and engineers are so important to working with customers and building real differentiations into products and services.”
With the proliferation of technologies like AI, machine learning and edge computing, the datasets that are causing CIOs headaches are only going to grow at an accelerated rate, making the need for effective DataOps more marked than ever before, Bowl believes.
“There is a big focus on AI and machine learning and that is only going to continue,” he concludes. “CIOs are spending all this money but not seeing much value. So how we build these environments for the future is going to be really important, I don’t think we’ll see just single repositories full of data.
“There is all this computing power at the edge, as well as public, private and hybrid cloud, which means a vast array of data. What will be really important is how you can do all of that integration and how you can catalogue and index all of that data in order to find it. Data is not going to be in one place and companies will need to holistically understand where their data is.”